Global Water Issue
“DaaS, 물 공익사업의 빅데이터 분석 한계 보완 가능”
(서비스형 데이터) (Water Utilities)
데이터 수집·정리·분석비용 알려져 있어 보다 수월한 예산계획 가능
선행 사례 연구서 긍정적 결과…못다 푼 문제 해결 위해 연구 지속
하·폐수 분야에 ‘서비스형 데이터(Data-as-a-Service, DaaS)’ 적용
Applying Data-as-a-Service To The Wastewater Sector
하·폐수 분야에서도 더 신뢰할 만한 정보 제공을 위해 서비스형 데이터를 보다 적극적으로 적용할 필요가 있다. 스마트워터 및 하·폐수 분야의 글로벌 허브인 스마트워터네트워크포럼(SWAN)의 아미르 칸(Amir Cahn) 상무이사는 물 전문 포털사이트 워터온라인(Water Online)에 지난 4월 6일자 기고를 통해 2017년 물연구재단(WRF) 연구의 일환으로 진행한 ‘세계 물 유틸리티들의 빅데이터 관리 관행’에 관한 설문조사 결과를 토대로 이 같이 강조했다. 그 내용을 번역했다.
실시간 모니터링 네트워크의 주요 관심사는 데이터의 정확성과 신뢰성이다. 지난 2017년 스마트워터네트워크포럼(Smart Water Networks Forum, SWAN)은 물연구재단(Water Research Foundation, WRF) 연구의 일환으로 전 세계 23개 물 유틸리티(Water Utilities)들의 빅데이터 분석 채택을 가로막는 요인을 비롯해 빅데이터(Big Data) 관리 관행을 조사했다. 결과는 다음과 같다.
설문조사 결과 ‘데이터 품질’과 ‘빅데이터를 지속적으로 처리하고 분석할 인재 부족’이 가장 큰 장애요인으로 확인되었다. ‘빅데이터를 구현할 인재 부족’과 ‘데이터 보안’이 그 뒤를 이었다. 이러한 취약점을 해결하기 위한 잠재적인 변화를 일으킬 수 있는 요인 중 하나는 데이터의 수집, 전달 및 검증에 대한 아웃소싱 접근방식인 ‘서비스형 데이터(Data-As-A-Service, DaaS)’ 모델이다.
A major concern with real-time monitoring networks is the accuracy and reliability of data. In 2017, the Smart Water Networks Forum(SWAN) surveyed 23 global water utilities about their Big Data management practices as part of a Water Research Foundation(WRF) study, including their barriers to adoption ― the results are shown below:
Based on these survey results, ‘Data quality’ and ‘Lack of talent to create and run Big Data processing and analytics on an ongoing basis’ were identified as the greatest impediment factors, followed by ‘Lack of talent to implement Big Data’ and ‘Data security’. One potential change agent to addressing these pain points is the ‘Data-as-a-Service’(DaaS) model, an outsourced approach to data collection, delivery, and verification.
DaaS란 무엇인가?
What Is DaaS?
DaaS는 요청 시 데이터에 접속해 조직의 데이터를 수집, 공유 및 해석하는 방식을 변화시킨 혁신적인 비즈니스 모델이다. 사르카(Sarkar) 박사는 그의 저서 『서비스형 데이터 : 재사용 가능한 엔터프라이즈 데이터 서비스를 제공하기 위한 프레임워크(Data as a Service: Framework for Providing Re-Usable Enterprise Data Services)』에서 DaaS를 ‘기업수준 표준(enterprise level standards)에 기초하여 설계된 재사용 가능한 데이터 서비스 세트를 설계하고 개발하기 위한 프레임워크’로 정의한다.
최근 몇 년간 DaaS는 모든 부문의 기업이 서비스 지향 아키텍처로 이동함에 따라 상당한 추진력을 얻었다. 본질적으로 DaaS는 사용자가 장비를 직접 구매하고 유지관리하는 대신 수신하고자 하는 최종 분석정보에 대해서만 비용을 지불하도록 한다. 따라서 하드웨어, 데이터 컬렉션, 스토리지 또는 데이터 제공업체에 남아있는 위험에 대한 지원에는 매몰 비용이 들지 않는다.
또한 DaaS는 직원 유지 및 교육과 관련된 장애물을 없애고 네트워크 운영 상태를 감독한다. 모든 서비스에 대한 위험을 관리하면서 고객에게 명확한 가치를 제공하고 달성하고자 하는 성공적인 결과를 촉진하는 것이 포함된다. [그림 1]은 DaaS의 주요 ‘서비스’ 측면을 보여준다.
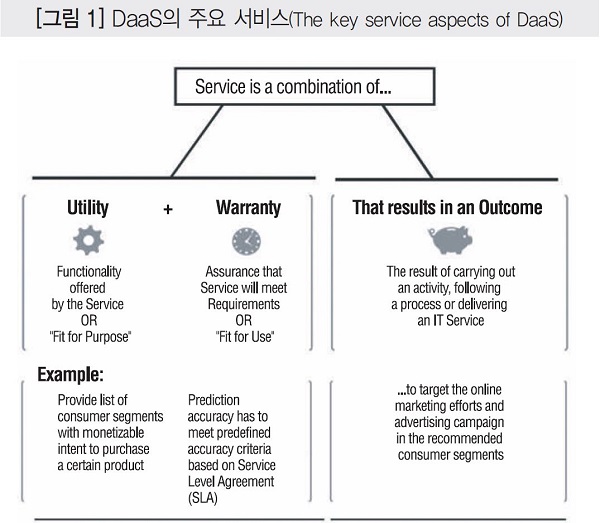
In recent years, DaaS has gained considerable momentum as enterprises across all sectors are moving towards a service-orientated architecture. In essence, DaaS enables users to only pay for the final analytics they wish to receive instead of purchasing and maintaining the equipment themselves. Thus, there are no sunk costs for hardware, data collection, storage, or support with these risks remaining with the data provider.
Additionally, DaaS relieves the obstacles involved in training and retaining staff to oversee the operational status of a network. Any type of service involves providing a clear value to customers and facilitating successful outcomes the customer wants to achieve, while managing associated risks. The below 2015 figure from Sarkar displays the key “service” aspects of DaaS:
스웨덴 함스타드대학교(Halmstad University)의 반 버트Maik van Vugt) 교수와 제이콥슨(Ole Jacobsen) 교수가 설명한대로 DaaS 과제에는 데이터 소유권, 데이터 보안 및 경제성에 대한 우려 등 주목할 만한 사안들도 있다. 그러나 DaaS의 주요 기능 중 하나는 데이터 수집, 정리 및 분석비용이 알려져 있어 예산 비용을 보다 쉽게 예측하고 미리 계획할 수 있다는 것이다.
사르카(Sarkar) 박사에 따르면, DaaS에는 다음과 같은 몇 가지 기본원칙이 존재한다.
·기술(테크놀로지)이 아닌 아키텍처 DaaS는 단순한 기술이나 애플리케이션을 넘어서는 아키텍처의 프레임워크(framework)다. 기본 기반은 일반적으로 서비스 재사용 개념에 기초하며, 사용자들이 웹(Web), 클라우드(Cloud) 및 관련 기술을 통해 공통의 표준화된 서비스를 조직 내에서 다목적 용도로 활용할 수 있도록 한다.
·데이터 품질에 중점 모든 DaaS 서비스 제공업체들에게 있어 게시된 데이터의 품질은 서비스 소비자가 단번에 알아차릴 수 있는 주요 전략 자산이다. 따라서 이것은 데이터 제공업체가 시장점유율을 높이기 위해 활용해야 하는 주요 차별화 요소로 간주되어야 한다. DaaS 가입자에게 제공되는 정보는 일관성 및 시의성, 정확성, 품질 및 사용적합성과 관련하여 비즈니스 이해관계자가 지정한 모든 서비스 수준 계약(Service-Level Agreements, SLA)을 충족해야 한다.
·데이터 거버넌스라는 도전과제 데이터 거버넌스는 종종 DaaS 프로그램의 가장 어려운 측면이다. 주요 거버넌스 문제에 대해 여러 이해관계자 간 합의를 얻기 위해서는 높은 수준 조정이 필요하기 때문이다. 이는 로컬(local) 데이터 법률(예: 데이터를 암호화해야 하는 경우), 데이터 품질 보증, 보안 및 개인 정보 보호 지원, 데이터 분류, 정보의 라이프사이클, 그리고 DaaS 시스템이 지원할 수 있는 감사 기능을 비롯한 여러 항목의 영향을 받는다. DaaS 프로그램을 고려하는 사람이라면 데이터 거버넌스가 조직 전체에 걸친 데이터 서비스의 장기적 성장과 지속가능성의 중요한 성공요소임을 알아야 한다.
As described by van Vugt and Jacobsen, there are also notable DaaS challenges, such as concerns over data ownership, data security, and affordability. However, one of the main features of DaaS is that the cost of data collection, cleansing, and analytics is known, making it easier to forecast budget expenses and plan ahead.
Below are a few guiding principles of DaaS, according to Sarkar.
·Architecture not technology DaaS is an architectural framework, beyond a mere technology or application. Its underlying foundation is typically based on the concept of service reuse, enabling users to utilize common, standardized services over the web, the cloud, and related technology for multiple purposes within an organization.
·Focus on data quality For any DaaS service provider, the quality of published data is the primary strategic asset that distinguishes them in the eyes of their service consumers. Therefore, it should be viewed as a key differentiator that must be exploited to drive market share by the data provider. The information fed to DaaS subscribers needs to be consistent, timely, and accurate and meet all the SLA (service-level agreements) specified by business stakeholders with regards to quality and fitness for use.
·Data governance challenge Data governance is often the most challenging aspect of a DaaS program due to the high degree of coordination required to gain consensus among multiple stakeholders on major governance issues. This is impacted by several items including local data laws (e.g., if the data must be encrypted), the support of data quality assurance, security and privacy compliance, data classification, information lifecycle, and auditing features that a DaaS system can support. Anyone considering a DaaS program should be aware that data governance is a critical success factor to the long-term growth and sustainability of data services across the organization.
물 분야에서 DaaS는 전체 하·폐수 시스템 운영, 합류식 하수관거 월류수(CSO) 모니터링, 수질 모니터링, 산업오염 탐지 등 다양한 서비스 운영 및 유지보수(O&M)를 외부 민간기업에 아웃소싱하는 유틸리티를 통해 하·폐수 관련 운영에 보다 보편적으로 적용되고 있다. 데이터 제공업체는 소비자에게 필요한 정보와 신뢰할 수 있는 결과를 제공하기 위해 데이터 측정, 수집, 저장 및 전송하는 데 필요한 장비를 도입하고 유지관리해야 할 책임이 있다.
다른 경우에, 유틸리티는 장비 자체(예: 유량 센서, 레벨 센서, 원격 스테이션)를 구매하거나, 장비를 임대하거나, 수신하고자 하는 데이터에 대해서만 비용을 지불하는 것 중에 선택할 수 있다. 물 분야의 비교적 새로운 비즈니스 모델인 DaaS가 유틸리티 운영에 영향을 미치고 고객 및 환경적 결과를 개선할 수 있는 방법에 대한 기존 정보는 제한적이다.
Within the water sector, DaaS is more commonly applied to wastewater operations with utilities outsourcing the operation and maintenance(O&M) of different services to outside private companies, such as for total wastewater system operations, combined sewage overflow(CSO) monitoring, water quality monitoring, and industrial pollution detection. To provide the required data, the data provider is responsible for acquiring and maintaining the necessary equipment to measure, collect, store, and transmit data to deliver reliable results.
In different cases, a utility may choose to purchase the equipment themselves (e.g., flow sensors, level sensors, remote stations), rent the equipment, or only pay for the data they wish to receive. As a relatively new business model for the water sector, there is limited existing information on how DaaS can impact utility operations and improve customer and environmental outcomes.
다음 단계
Next Steps
하·폐수 분야에서 DaaS에 대한 여러 유틸리티 사례연구(utility case study)가 성공했음에도 불구하고 다음과 같이 여전히 밝혀지지 않은 것들이 있다.
·DaaS를 구현하기 위한 주요 유틸리티 동기는 무엇인가? 가장 큰 장벽은 무엇인가?
·DaaS는 자체 네트워크를 설치, 운영 및 유지관리할 수 있는 능력이 없는 유틸리티에만 적합한가?
·DaaS 유틸리티 관행은 서로 다른 하·폐수 애플리케이션과 여러 부문에서 어떻게 비교되는가?
·DaaS는 유틸리티 운영관리(O&M) 효율성을 어떻게 향상시키는가?
·DaaS 유틸리티들은 DaaS가 아닌 유틸리티에 비해 규제 준수(regulatory compliance)가 개선되었는가?
·DaaS 유틸리티들은 데이터, 보고서 요약 또는 예측 인사이트(Predictive insight)를 얻는 것을 선호하는가?
·무엇이 DaaS를 유틸리티에 적합하게 만드는가?
앞으로 6∼9개월 동안 필자는 이러한 질문들에 대한 답을 찾기 위해 (1)글로벌 유틸리티 조사와 (2)최상의 데이터 관리 전략, 데이터 품질 절차, 계약 환경 설정, 규제 시행 등을 결정하기 위해 다양한 하·폐수 애플리케이션 분야서 DaaS를 사용하는 일부 유틸리티에 대한 심층적인 연구를 수행할 것이다. 이러한 각각의 연구는 스마트워터네트워크포럼(SWAN) 회원들에게 무료로 제공된다.
여기에 대한 자세한 내용이 필요하거나 연구에 참여를 희망하는 사람은 필자(아미르 칸 박사)에게 메일(amirc@swan-forum.com)로 문의하면 된다.
Despite several successful utility case studies for DaaS within the wastewater sector, there are still several unknowns, such as the questions listed below.
·What are the main utility motivations to implement DaaS? What are the biggest barriers?
·Is DaaS only suitable for utilities that do not have the capacity to install, operate, and maintain their own network?
·How do utility DaaS practices compare across different wastewater applications, as well as across different sectors?
·How does DaaS improve the efficiency of utility O&M?
·Do DaaS utilities have improved regulatory compliance compared to non-DaaS utilities?
·Do DaaS utilities prefer to just acquire data, report summaries, or also predictive insights?
·What makes a utility an appropriate fit for DaaS?
Over the course of the next 6 to 9 months, I will investigate these questions by carrying out (1) a global utility survey and (2) an in-depth study of select utilities using DaaS for different wastewater applications to determine best data management strategies, data quality procedures, contract preferences, regulatory performance, and more. Each of these studies will be available for free to SWAN members.
For more information and to actively participate in this research, please contact Amir Cahn at amirc@swan-forum.com.
저자소개 아미르 칸(Amir Cahn) 박사는 스마트워터 및 하·폐수 분야를 선도하는 글로벌 허브인 스마트워터 네트워크 포럼(Smart Water Networks Forum, SWAN)의 상임이사다. 스마트워터네트워크포럼은 전 세계 물 및 하·폐수 네트워크에서 데이터 중심 기술의 사용을 장려하는 글로벌 비영리단체다. 영국에 기반을 둔 스마트워터네트워크포럼은 세계적인 수자원 유틸리티 및 솔루션 제공업체, 학계·연구계 관계자, 투자기관 및 규제기관, 기타 산업 전문가들을 한 데 모아 수자원 및 하·폐수 분야에 ‘스마트(smart)’한 데이터 중심 솔루션의 채택을 가속화하기 위해 노력한다. 포럼에 대한 자세한 내용은 홈페이지(http://www.swan-forum.com)에서 확인할 수 있다. |
[출처 = Water Online(https://www.wateronline.com/doc/applying-data-as-a-service-to-the-wastewater-sector-0001) / 2020년 4월 6일자]
[『워터저널』 2020년 5월호에 게재]